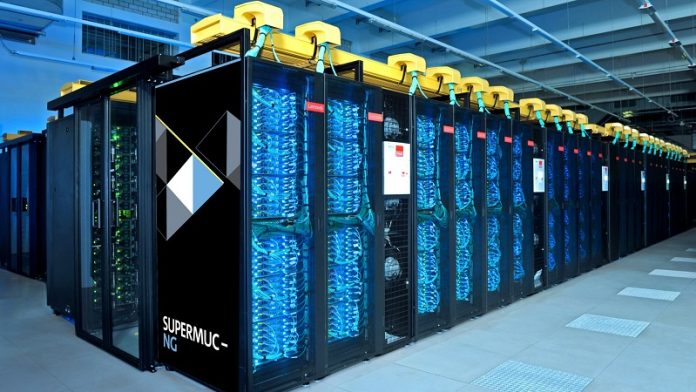
The SuperMUC-NG at the Leibniz Supercomputing Centre is the eighth fastest computer in the world. Credit: Veronika Hohenegger, LRZ.
Artificial intelligence (AI) has become a key part of our daily lives, powering everything from chatbots to image recognition.
However, training AI models requires enormous amounts of energy, mostly supplied by data centers.
In Germany alone, data centers used about 16 billion kilowatt-hours (kWh) of electricity in 2020—about 1% of the country’s total energy consumption.
By 2025, this number is expected to rise to 22 billion kWh as AI models become even more complex.
To tackle this growing energy demand, researchers at the Technical University of Munich (TUM) have developed a new AI training method that is 100 times faster than existing techniques.
This breakthrough could dramatically cut the energy required for AI model training while maintaining high accuracy.
The team presented their findings at the Neural Information Processing Systems (NeurIPS) conference in Vancouver, held from December 10 to 15, 2024.
AI models, like human brains, use networks of interconnected nodes called artificial neurons. These neurons process data by assigning different weights to input signals and passing them through the network.
Training an AI model involves adjusting these weights over many iterations, which requires a huge amount of computing power and electricity.
Traditionally, AI training starts with random values for these weights, which are gradually refined to improve accuracy. However, this process is slow and energy-intensive.
Professor Felix Dietrich and his team at TUM have created a new training method that uses probability-based learning instead of repeated adjustments.
Instead of adjusting all parameters over multiple rounds, their method focuses on selecting key values at critical points in the data—where significant changes happen.
This new approach is particularly useful for dynamic systems, such as climate models or financial market predictions, where data changes over time.
“Our method determines the necessary parameters with minimal computing power,” says Dietrich. “This makes AI training much faster and much more energy-efficient. At the same time, we’ve found that the accuracy of our method is just as good as traditional training approaches.”
This new training method could play a major role in reducing the environmental impact of AI. As AI continues to evolve and expand, making training more energy-efficient will be essential. With this breakthrough, the future of AI could be both smarter and greener.