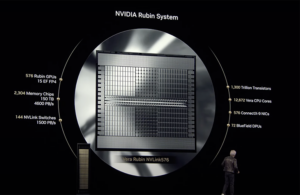
Vera Rubin
The term “[exascale computing](https://www.rdworldonline.com/an-overview-of-the-late-2024-supercomputing-landscape-in-6-charts/)” used to conjure images of sprawling supercomputer facilities like Frontier or El Capitan. Now, NVIDIA is attaching that same term to a single rack of its upcoming Rubin Ultra AI chips. Scheduled for 2027, Rubin Ultra is projected to deliver 15 exaflops of AI compute. That computational horsepower, in a sense, would rival the throughput of today’s largest supercomputers, at least in lower-precision AI tasks.
But the comparison between future-gen GPUs and current-gen supercomputers is decidedly apples to oranges. Before picturing an entire national lab supercomputer replaced by one server cabinet, there’s a crucial asterisk: NVIDIA’s exaflops claims refer to AI-oriented precision, not the 64-bit performance that defines top supercomputers. Still, for large-scale inference and training, a Rubin Ultra rack represents a seismic shift.
### Blackwell to Feynman: A multi-year GPU architecture roadmap
During his GTC 2025 keynote, CEO Jensen Huang unveiled a plan for powering what he calls the world’s “AI factories.” It begins with Blackwell Ultra in the second half of 2025, offering “one and a half times more FLOPS” and “two times more networking bandwidth” compared to the current Hopper architecture. The roadmap consists of the following:
* Vera Rubin (H2 2026): New CPU, new GPU, new CX9 networking, and HBM4 memory.
* Vera Rubin Ultra (H2 2027): A jump to 15 exaFLOPS of AI compute with bandwidth (4,600 TB/s).
* Feynman (2028): The next-generation platform with details yet to come.
### From data centers to ‘AI factories’
Huang explained the long planning horizons for such undertakings: “Look, we’re building AI factories and AI infrastructure. It’s going to take years of planning… which is the reason why I show you our roadmap a couple two, three years in advance so that we don’t surprise you.”
NVIDIA sees a paradigm shift from conventional data centers to “AI factories,” where the primary output is “tokens” for generative AI services. Huang described this transition: “The computer has become a generator of tokens, not a retrieval of files… “
> I call them AI factories because they have one job and one job only: generating these incredible tokens that we then reconstitute into music, into words, into videos, into research, into chemicals or proteins.
>
> Jensen Huang
Supporting these AI factories is NVIDIA Dynamo—an “operating system” tailored for generative AI infrastructure, effectively replacing older enterprise software stacks like VMware. Dynamo OS goes beyond just managing hardware resources—it orchestrates complex AI workloads, dynamically partitions GPUs for different phases of inference (prefill and decode), and manages key-value caches critical for large language models. As Huang described it, Dynamo is “the operating system of the AI factory,” analogous to the original Dynamo that powered the industrial revolution.
### Networking for scale could help tame soaring inference demands
Another shift in the new architectures is the move to disaggregated MVLink and co-packaged silicon photonics. These technologies overcome the limitations of integrated MVLink and enable dramatic power efficiency gains compared to traditional transceivers. This architectural shift is what allows NVIDIA to scale systems to potentially connect millions of GPUs while maintaining the bandwidth necessary for massive AI models.
As agentic AI systems grow more complex, inference is emerging as a major driver of computation: “The amount of computation we need at this point… is easily 100 times more than we thought we needed this time last year.”
While DeepSeek’s rise was a momentary headache for NVIDIA, Huang uses its R1 model to make a point. A simple task of asking a traditional large language model to help with, say, a seating arrangement might use just over 400 tokens. But R1 could use more than 8,000 tokens of “thinking” on the same task while coming up with a more satisfactory answer. Roughly 20x more tokens and well more than 100x more compute in this example.
The amount of compute is steadily going up, necessitating new architectures to handle the burgeoning demand. “Once a year, once a year like clock ticks, once a year,” Huang said. “We try to take silicon risk or networking risk or system chassis risk in pieces so that we can move the industry forward.”