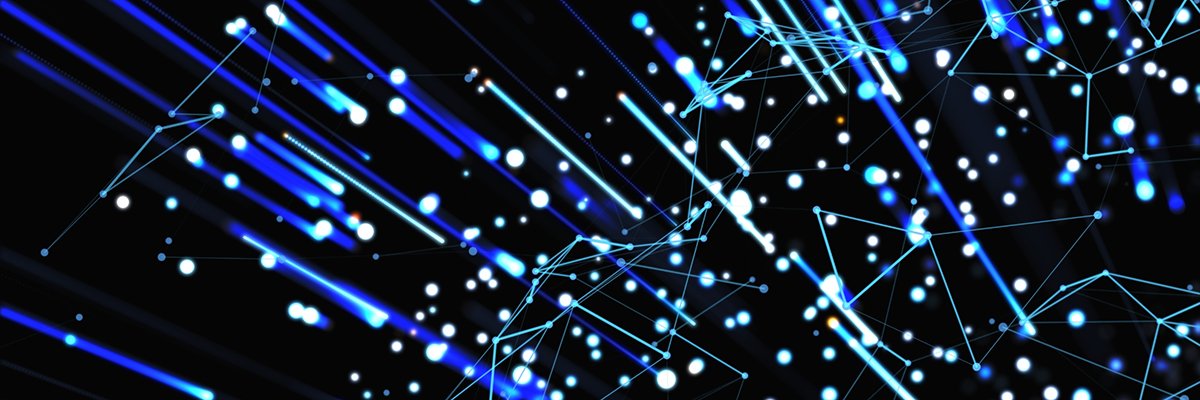
Keysight Technologies has launched Keysight AI (KAI) Data Centre Builder, a software suite designed to emulate real-world workloads to evaluate how new algorithms, components and protocols impact the performance of artificial intelligence (AI) training.
Fundamentally, the workload emulation capabilities of the KAI Data Centre Builder are attributed with enabling AI operators, graphics processing unit (GPU) cloud providers and infrastructure suppliers to bring realistic AI workloads into their lab setups to validate the evolving designs of AI clusters and new components. They are said to be able to experiment to fine-tune model partitioning schemas, parameters and algorithms to optimise the infrastructure and improve AI workload performance.
Behind the KAI Data Centre Builder’s operation is the fact that AI operators use various parallel processing strategies, such as model partitioning, to accelerate AI model training. Aligning model partitioning with AI cluster topology and configuration enhances training performance. Keysight Technologies said that during the AI cluster design phase, critical questions are best answered through experimentation, with many focused on data movement efficiency between the GPUs.
Key considerations include scale-up design of GPU interconnects inside an AI host or rack; scale-out network design, including bandwidth per GPU and topology; configuration of network load balancing and congestion control; and tuning of the training framework parameters.
KAI Data Centre Builder’s workload emulation capability is built to integrate large language model (LLM) and other AI model training workloads into the design and validation of AI infrastructure components, in particular networks. It’s intended to enable tighter synergy between hardware design, protocols, architectures and AI training algorithms, boosting system performance.
The workload emulation service reproduces network communication patterns of real-world AI training jobs to accelerate experimentation, reduce the learning curve necessary for proficiency and provide deeper insights into the cause of performance degradation, which is not easily attainable by experimenting with real AI training jobs.
The result, said Keysight, is that its customers can access a library of LLM workloads such as GPT and Llama, with a selection of popular model partitioning schemas like data parallel (DP), fully sharded data parallel (FSDP) and three-dimensional (3D) parallelism.
The company added that using the workload emulation application in the KAI Data Centre Builder enabled AI operators to experiment with parallelism parameters, including partition sizes and their distribution over the available AI infrastructure (scheduling) and understand the impact of communications in and among partitions on overall job completion time (JCT). It’s also attributed with allowing users to identify low-performing collective operation, and drill down to identify bottlenecks and analyse network utilisation, tail latency, and congestion to understand the impact they have on JCT.
“As AI infrastructure grows in scale and complexity, the need for full-stack validation and optimisation becomes crucial,” said Ram Periakaruppan, vice-president and general manager of network test and security solutions at Keysight.
“To avoid costly delays and rework, it’s essential to shift validation to earlier phases of the design and manufacturing cycle. KAI Data Center Builder’s workload emulation brings a new level of realism to AI component and system design, optimising workloads for peak performance.”